Federated Learning in Autonomous Vehicles: Driving the Future of Mobility
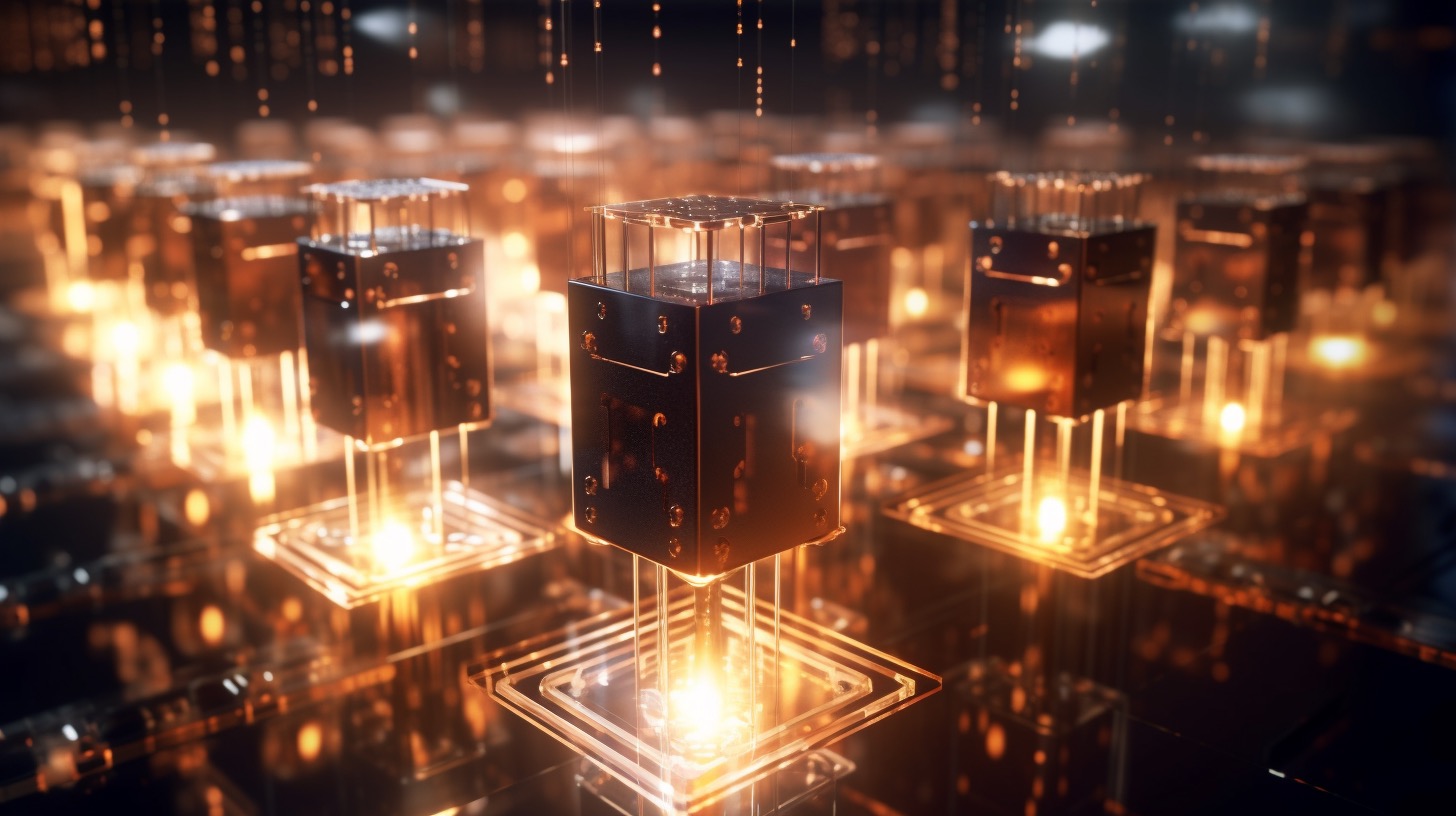
Federated Learning in Autonomous Vehicles: Driving the Future of Mobility
Federated learning is an innovative approach to machine learning that is poised to revolutionize the way autonomous vehicles learn and adapt to their environments. This decentralized method of training algorithms allows for the sharing of data and insights without compromising privacy or security, making it an ideal solution for the connected world of self-driving cars. As the automotive industry moves toward a future of increased autonomy, federated learning will play a critical role in driving the development of smarter, safer, and more efficient vehicles.
The concept of federated learning was first introduced by Google in 2017 as a way to train machine learning models on decentralized data. Instead of collecting and centralizing data from multiple sources, federated learning allows algorithms to be trained on local devices, such as smartphones or vehicles, using their own data. The insights gained from this local training are then shared with a central server, which aggregates the information and updates the global model. This process is repeated across all participating devices, resulting in a more accurate and robust model that benefits from the collective intelligence of the entire network.
One of the key advantages of federated learning is its ability to protect privacy and security. Since data remains on local devices and is not transmitted to a central server, there is a reduced risk of data breaches or unauthorized access. This is particularly important in the context of autonomous vehicles, where sensitive information about driving habits, locations, and personal preferences could be exploited by malicious actors. By keeping data local and only sharing insights, federated learning enables vehicles to learn from each other while maintaining the privacy of their users.
Another significant benefit of federated learning is its potential to improve the performance and efficiency of autonomous vehicles. Traditional machine learning models are often trained on large, centralized datasets that may not accurately represent the diverse range of driving conditions and scenarios encountered by vehicles in the real world. Federated learning, on the other hand, allows vehicles to learn from their own experiences and those of their peers, resulting in a more comprehensive understanding of the environment. This can lead to improved decision-making, faster adaptation to new situations, and ultimately, safer and more efficient driving.
In addition to these benefits, federated learning also has the potential to accelerate the development and deployment of autonomous vehicles. By enabling vehicles to learn from each other in real-time, federated learning can help to overcome some of the limitations of traditional machine learning methods, such as the need for large amounts of labeled data and the time-consuming process of training and updating models. This can result in faster innovation and more rapid progress toward the widespread adoption of self-driving cars.
Several major players in the automotive and technology industries are already exploring the potential of federated learning in the context of autonomous vehicles. For example, BMW and Microsoft recently announced a partnership to develop an open-source platform for federated learning, which will enable vehicles to share data and insights without compromising privacy. Similarly, Chinese tech giant Baidu has launched a federated learning platform for its Apollo autonomous driving project, aiming to improve the performance and safety of its self-driving cars.
In conclusion, federated learning represents a promising new approach to machine learning that has the potential to drive significant advancements in the field of autonomous vehicles. By enabling vehicles to learn from each other while protecting privacy and security, federated learning can help to create smarter, safer, and more efficient transportation systems for the future. As the automotive industry continues to embrace this innovative technology, it is clear that federated learning will play a critical role in shaping the future of mobility.